The National Association of Mutual Insurance Companies (NAMIC), a United States insurance body whose membership includes national and regional mutual insurance companies, says that attempts to apply general AI regulations to the insurance industry could make it more difficult to price risks appropriately.
Background
Regulators are increasingly implementing policies to prevent discrimination through AI use across a number of sectors.
NAMIC reports that 18 states in the United States are considering AI bills which are “overly broad”, and that state insurance departments continue to adopt the National Association of Insurance Commissioners’ Model Bulletin on the Use of Artificial Intelligence Systems by Insurers. Both implement standards around algorithmic bias, which NAMIC describes as “nebulous”.
What has NAMIC said?
NAMIC notes that insurance and the process of risk-based pricing are highly susceptible to any regulations which restrict an insurer’s ability to accurately predict the rate of loss by policyholders. As risk-based pricing is a data-driven exercise, it benefits from increased precision through leveraging big data and AI.
One key concern with the use of AI is the risk of proxy discrimination. This is where data elements, which are strongly correlated to risk, can also be strongly correlated with protected characteristics. AI advancements allow for greater insights, which gives rise to increased concerns of ‘algorithmic bias’ which can in turn adversely impact insurance availability and affordability for some vulnerable or disadvantaged customers. NAMIC argues that policyholders may be harmed by growing efforts to elevate concepts of ‘fairness’ where this is divorced from actuarial science.
Lindsey Klarkowski, NAMIC Vice President, says the real issue is not algorithmic bias but “the existence of elevated risk representation in a group,” and that “rather than discussing the artificial insurance pricing, we should all be asking how best to mitigate those underlying risks that are represented in a particular risk class”.
1. Insurance is a unique product
NAMIC explains that insurance is often grouped with other industries in policy conversations and legislative and regulatory proposals, particularly related to discrimination through AI and algorithmic bias.
However, insurance is a unique product which should be regulated distinctly. It is not like other products which customers pick off the shelf, as the price of insurance is not determined based on known costs, expenses, and profits. The actual cost to insurers is unknown at the time of the sale. Further, “Insurance classifies based on risk and insurance law requires those risk classifications to be actuarially sound and not unfairly discriminatory”.
2. The use of AI can enable widening availability of insurance to more customers
Countering arguments that AI may create a “risk pool of one”; causing accessibility issues for high-risk insurers, Klarkowski says that without group probabilities it is impossible to set insurance prices. Competition between insurers drives refinement of risk pools, and more accurate risk classification means that premiums are more closely aligned with risk. This drives lower risk insureds to insurers with more refined risk classifications and low corresponding premiums.
NAMIC argues that a competitive market for insurance is the most effective guarantor of low prices and widespread availability. It also incentivises customer risk reduction.
Risk spreading is essential to insurers, meaning that there cannot be a ‘risk pool of one’. This is because risk spreading is only accomplished if there are large numbers of insureds. Insurers need to spread risk across insureds, as no insured is entirely without risk. This means that insurance is only possible due to risk pooling. Insurers “must utilize a risk classification system that will allow it to offer insurance to as many potential customers as possible.” (Detlefsen, Robert, Ph.D. The Case for Underwriting Freedom: How Competitive Risk Analysis Promotes Fairness and Efficiency in Property/Casualty Insurance Markets).
Klarkowski argues that “If an insurer can more accurately assess a risk, it can more accurately discern whether it can absorb a higher risk insured. This fact, coupled with the economic drivers of market penetration, results in increased availability of insurance, even for high-risk individuals”.
3. Fairness for customers means matching rates to risk, not mandating one price for all
NAMIC asserts that one equal price for all insurance customers is not fair. Restrictions on insurers’ ability to price risk may result in more, rather than fewer, access and affordability issues. Karkowski says that the level of risk presented by a customer is not necessarily correlated with their level of wealth. This means the regulations which redistribute risk may have unintended consequence. For example, it could result in a less affluent insured with low risk subsidising an affluent customer with a much higher risk. As an example, a middle-class worker driving a Toyota SUV could end up subsidising a multi-millionaire driving a high-ed super car. It is important for insurers to be able to differentiate between risks. Forcing less risky customers to subsidise riskier customers causes market distortions, which can affect availability and affordability.
4. Outcomes-based testing methods for algorithmic bias are unreliable and incompatible with insurance regulations
Outcomes-based testing for algorithmic bias in insurance is where the focus is on evaluating the actual results produced by a model, rather than the model’s internal workings. It involves comparing outcomes across different demographics to identify whether groups are treated ‘unfairly’, even if the model’s internal workings and logic is fair.
Insurance law in various areas of the world (including some US states) recognises that the ability to discern between different risks is essential to insurance, and specifically only prohibits unfair discrimination. Prohibiting risk-rating factors which are predictive of risk, but which result in higher premiums for protected classes opposes risk-based pricing. As premiums would no longer be based on underlying insurance costs, this would unfairly discriminate against some customers. NAMIC predicts that if premiums were allowed to diverge from risk, this would result in a bad outcome for some insurance customers and disrupt the insurance market.
5. There are inherent limitations in reliable demographic data and testing methodologies with outcomes-based testing methods in insurance
NAMIC points to inherent limitations in reliable demographic data for the purposes of testing, and in reliable testing methodologies for the purpose of identifying any bias or disparate impact. Many insurers do not collect or use protected class data, and in some places the collection of such data is prohibited. This means that analysis of protected classes would require estimating of customers’ protected class status. NAMIC asserts that this is unreliable, particularly for some protected characteristics such as religion or sexual orientation.
NAMIC also argues that the available testing methodologies are flawed. For example, using control variables takes predictive power away from factors that are already in the model. This can result in inaccurate pricing.
Conclusion
Fairness in insurance is achieved by accurately matching price to a policyholder’s risk, based on sound actuarial principles.
NAMIC raises a number of interesting points about the importance of accurately matching price to customer risk, and the implications of broader AI anti-discrimination regulations to the unique considerations faced by insurers.
The balance between pricing according to risk and discrimination is a hot topic, and one which is likely to be subject to further regulatory scrutiny. The debate over what factors are ‘fair’ to use in insurance models is likely to accelerate with the use of artificial intelligence.
For more discussion on this interesting topic, please see our other articles on AI, underwriting, and the actuarial defence:
AI putting the ‘actuarial defence’ to the test?
AI in insurance: Targeted marketing as a quasi-underwriting function
Contents
- The Word, April 2025
- The impact of Martyn’s Law for insurers
- Redefining standards: A spotlight on Which?'s call for fairer insurance definitions
- Addressing insurance fraud: Aviva's response and industry-wide implications
- The Contracts of Insurance Act 2024 in New Zealand: What will this mean for insurers?
- Insurance as a force for good: Protecting domestic violence victims
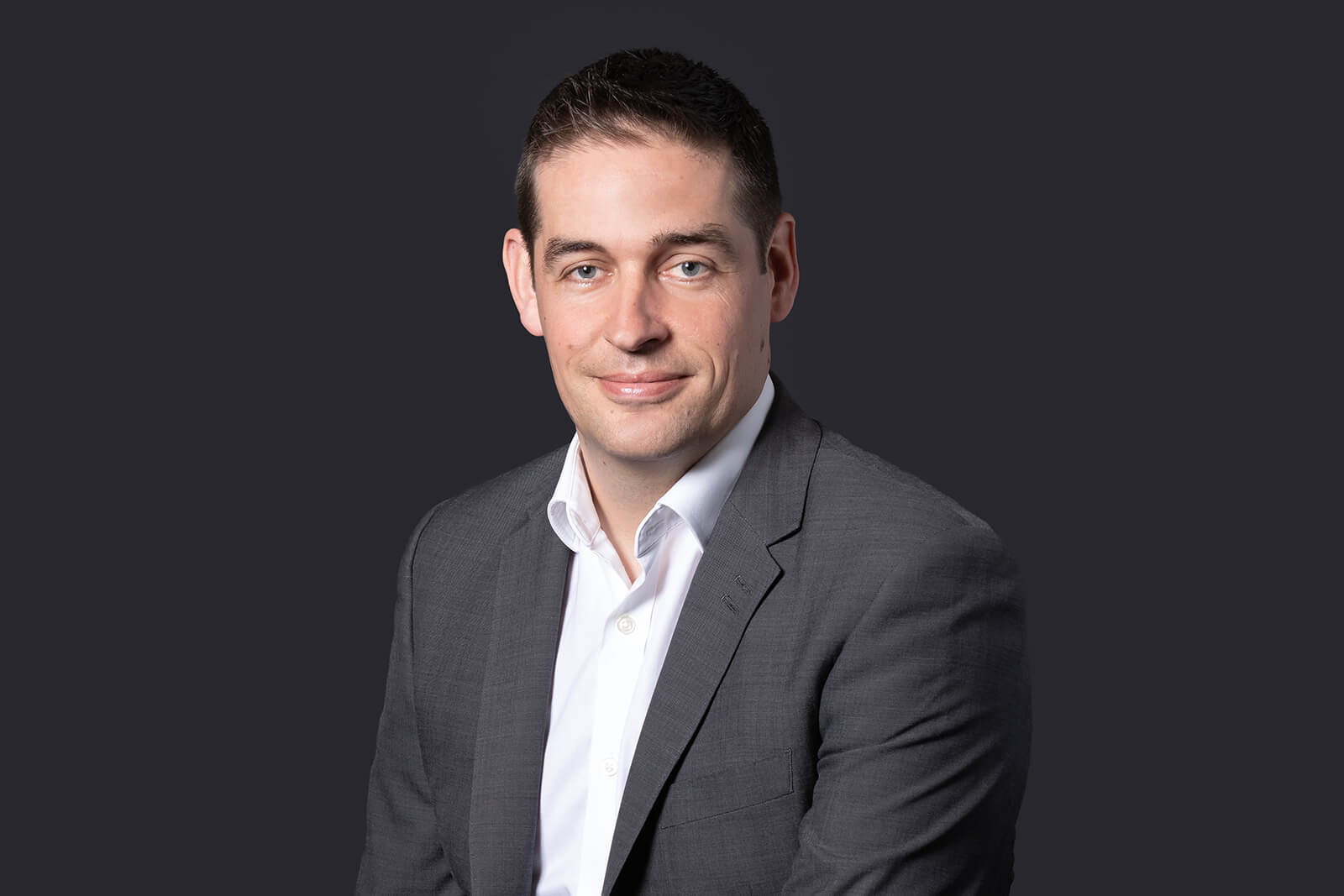
Tim Johnson
Partner
tim.johnson@brownejacobson.com
+44 (0)115 976 6557